Applying transfer learning for improved binary seizure detection accuracy.
Transfer learning for Health Characteristics Prediction
Recent advances in phenotypic information and machine learning have significantly improved binary seizure detection accuracy. However, classifying specific seizure types remains challenging due to limited annotated data. Transfer learning shows promise in overcoming data scarcity for better accuracy on smaller datasets.
Yet, selecting the ideal transferable model for a given dataset is complex and repetitive, and a single model may not capture all input data features effectively. Additionally, genotypic data is often overlooked in previous AI-based seizure detection studies, missing potential insights into genetic predispositions.
To address these challenges, we propose a seizure-type classification framework (Figure 1) with a multi-model weighting system to streamline computation and reduce processing time.
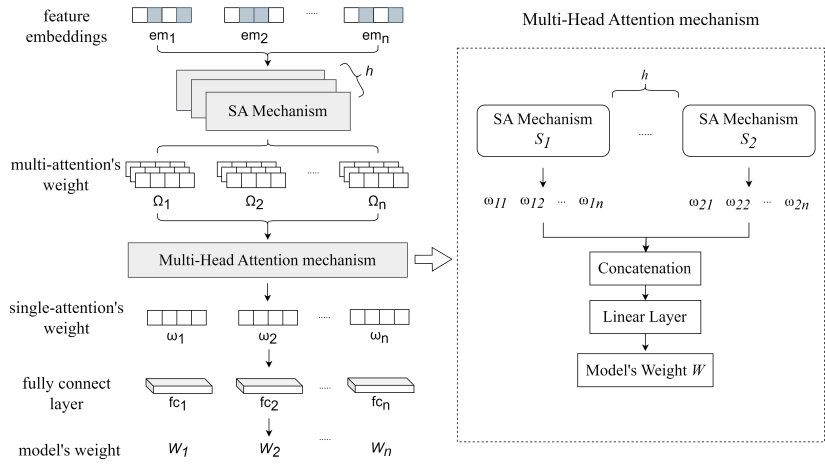
Figure 1: The multi-model weighting system consists of multiple paralleled SA mechanisms.
We also conduct Polygenic Risk Score (PRS) analysis to bridge the gap between genotypic and phenotypic data, enhancing seizure detection comprehensiveness and precision. Our model outperforms similar classifiers by 13-16% on the Temple University Hospital EEG Seizure Corpus dataset. This study pioneers the use of a multi-source transfer learning framework in type-specific seizure classification. Read the full paper (forthcoming).
This paper presents contributions to the field of seizure-type classification, focusing on improving classification accuracy and incorporating genetic insights.
Four key highlights delineate the innovative approaches used in this study:
- Reducing Channel Requirements: The research successfully minimized the number of EEG channels needed for seizure classification, from 22 down to just 4. This achievement was made possible by harnessing EEG effective connectivity features. The classifier's exceptional performance demonstrates the effectiveness of these 4-channel connectivity features in capturing the brain's structural relationships during epileptic seizures.
- Multi-Model Transfer Learning: This study breaks proposed a multi-source domain adaptation method for type-specific seizure classification. Employing a multi-model-based transfer learning approach, the researchers utilized transferable information to enhance overall classification performance. This novel approach promises to reshape the seizure classification landscape by utilizing diverse data sources.
- Self-Attention Mechanism: A self-attention (SA) mechanism was developed to assign weights to each model, facilitating the identification of crucial information from various models. The resulting classifier, constructed by combining pre-trained networks, outperformed conventional machine learning classifiers. This innovative approach improves model interpretability and performance, ushering in a new era of seizure classification.
- Genotypic Integration with PRS Analysis: To enrich phenotypic data with genetic insights, the researchers integrated Polygenic Risk Score (PRS) analysis into seizure detection. This integration bridges the divide between genetic predisposition and clinical presentation, offering a comprehensive understanding of epilepsy's etiology. This innovative approach opens doors to personalized medicine in epilepsy management.