Our research team applies advanced AI techniques, unlocking human genomes in order to better tackle the critical global problem posed by chronic diseases.
Research Trajectories
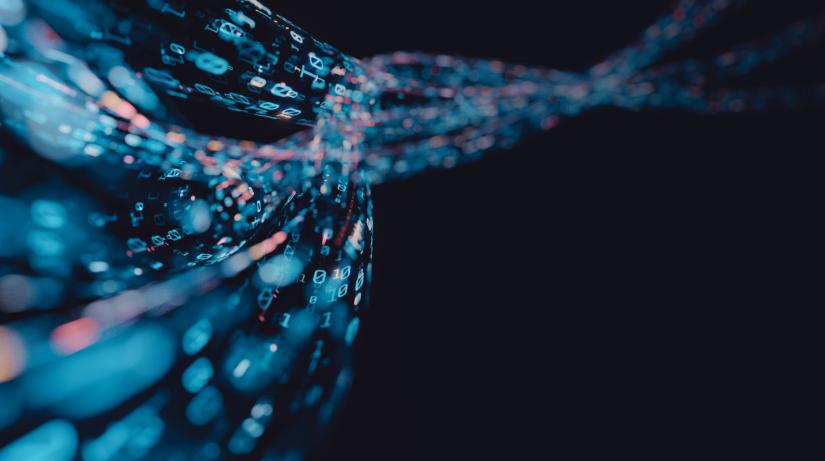
Better Understanding Stroke: A New Research Approach
Why This Matters: Stroke is a major health issue worldwide. Early detection is crucial as it can significantly improve recovery outcomes. Typically, stroke prediction is based on a person’s medical history and lifestyle, but genetic information also plays a crucial role. This genetic insight is vital not just for predicting the likelihood of a stroke but also for understanding its various types, which can guide doctors in choosing the right treatment plans.
Our Research: We believe that genetic information (genotypes) provides deeper insights into the different types of strokes, beyond what traditional risk factors can offer. This is particularly true for strokes with unknown causes, which account for about 30 - 40% of cases. Our approach uses advanced AI to analyse whole genome sequencing data, aiming to discover new genetic markers that could revolutionise stroke understanding and treatment.
How We Did It: Our team developed a specialised AI model, tailored to stroke prediction, that simplifies complex genetic data into a more manageable form (a process we call 'embedding'). Using a technique known as contrastive learning, the model more effectively identifies individuals at risk of a stroke. It also takes into account key health factors like blood pressure and BMI. By integrating genetic data with these health indicators, our model achieves greater accuracy in predicting various stroke types.
What We Found: Our model, when tested against the UK Biobank dataset—one of the world’s largest health data collections—outperformed existing methods in predicting stroke risk. It identifies 459 genomic locations that are linked to various stroke types, providing a way to measure an individual's future risk of stroke. These findings are particularly crucial for strokes with unclear causes. This research highlights the importance of genetics in understanding complex diseases like stroke and suggests the potential applicability of this method to other medical conditions with indeterminate origins.
Tackling Chronic Diseases with Advanced AI Techniques and Human Genomes
Understanding the Challenge: Chronic diseases like diabetes, hypertension, and hypercholesterolemia affect millions globally. Identifying these conditions early and managing them effectively is a major challenge in healthcare. Traditional methods for predicting the risk of these diseases have their limitations, accuracy and practicality still need to be improved.
Our Innovative AI Solutions: We have developed two cutting-edge AI algorithms: The Genome-based Graph Convolutional Network (Geno-GCN) and the Enhanced Indel Deep Neural Network (EI-DNN). Geno-GCN is specially designed to analyse genetic data for predicting diabetes risk, focusing on both positive and negative genetic influences. EI-DNN, on the other hand, looks at a broader range of genetic data, including indels and SNPs, to enhance disease prediction.
Impactful Results: When tested on Australia's largest genome bank, Geno-GCN outdid traditional methods and other AI techniques in accuracy and reliability, especially in personalising diabetes risk prediction. EI-DNN also showed great results, particularly in predicting hypertension and hypercholesterolemia. These models simplify risk assessment, reduce healthcare professionals' workload, and pave the way for tailored disease prevention strategies in personalised medicine.
Improving Stroke Treatment with Genetic Insights
The Clinical Issue: Stroke is a leading cause of death, and treatments often involve drugs (thrombolytics) to dissolve blood clots in the brain. However, there is a risk of haemorrhagic transformation (HT) - a severe side effect that can lead to disability or death. Understanding how genetic factors affect patient responses to thrombolytic drugs like Alteplase and Tenecteplase is key to reducing HT risk.
Our AI-Driven Approach: We have combined whole genome sequencing, AI, and pharmacogenomics to develop a novel approach. This includes using AI to recommend top gene lists, a graph neural network to study genetic variants and drug metabolism, and expert compilation of gene lists. This method blends AI analysis with expert human insights, exploring how genetics influence the effectiveness and safety of thrombolytic drugs.
Valuable Outcomes: We created a comprehensive gene panel of 30 genes, which could be a game-changer for clinicians in choosing the right thrombolytic drug and minimising HT risk. This gene panel could be used in acute ischemic stroke treatment protocols, allowing clinicians to perform a simple blood test to identify genetic variants associated with HT. This can help formulate safer treatment plans with a lower risk of serious bleeding events.
BiblioEngine: An AI-empowered Research Tool for Paper Reading and Analysis
Paper Reading vs. Explosive Literature: The rapidly growing scientific literature presents a critical challenge for biomedical and clinical researchers who need to keep pace with emerging novel knowledge and understand the changing research landscape. As indicated by PubMed, the current volume of biomedical publications, which is more than 36 million, hinders scholars, especially early-career researchers, from viewing global research from a bird-eye view and absorbing emerging knowledge efficiently.
What Is BiblioEngine: Recognising this need, our team leveraged the power of artificial intelligence (AI) and developed a powerful research tool – BiblioEngine. As an AI-driven tool, it can deliver distilled research evidence and insights via automatic large-scale literature analysis, further facilitating research landscape profiling and latent knowledge discovery.
What Can BiblioEngine Do: Building upon the integration of multiple AI-empowered data sources, BiblioEngine incorporates four core AI algorithmic modules: a scientific evolutionary pathway (SEP) module that presents how research topics evolve, an NLP module that extracts knowledge units from scientific text, a network analysis module that unravels knowledge unit associations, a recommender system module that suggests potential research collaborators and fields.
A Powerful Spur for 23Strands: BiblioEngine contributed to unparalleled efficiency improvements for evidence-based, genomics-informed reporting at our industry partner, 23Strands. In the past, a skilled researcher needed approximately four weeks to generate a genomic-informed report due to the time and human effort required for the manual investigation of 100-150 research papers. This traditional workflow was simply impossible for large-scale literature data analysis even without stretched human resources. The incorporation of BiblioEngine has now revolutionized this process to a couple of hours, with data-driven insights from thousands of relevant research papers automatically summarised in intuitive tables and visualisations and readable for researchers and clinicians. The reduction in time frees up valuable human resources for more strategic and creative endeavours. Real-world case studies have demonstrated its successful application in atrial fibrillation, COVID-19, and stroke.