Online Seminar: Dr Markus Heyl, Max Planck Inst., Germany
Machine learning quantum circuits for digital quantum simulation
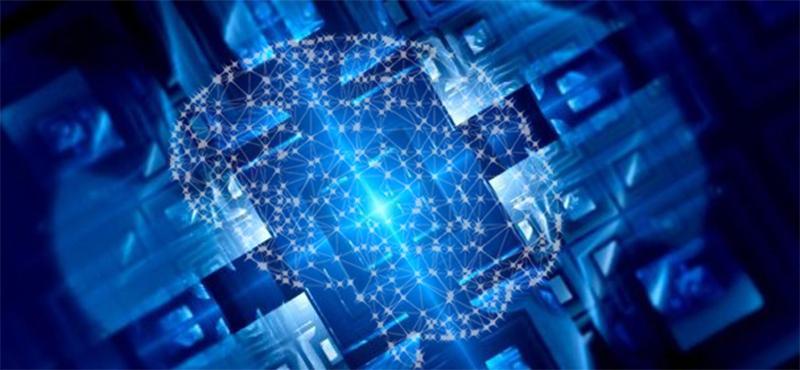
Reinforcement Learning for Digital Quantum Simulation
SPEAKER: Dr Markus Heyl
AFFILIATION: Max Planck Institute of Quantum Optics, Dresden
HOSTED BY: A/Prof Nathan Langford, UTS Centre for Quantum Software and Information
ABSTRACT:
Digital quantum simulation is a promising application for quantum computers. Their free programmability provides the potential to simulate the unitary evolution of any many-body Hamiltonian with bounded spectrum by discretizing the time evolution operator through a sequence of elementary quantum gates, typically achieved using Trotterization.
A fundamental challenge in this context originates from experimental imperfections for the involved quantum gates, which critically limits the number of attainable gates within a reasonable accuracy and therefore the achievable system sizes and simulation times.
In this talk, I will introduce a reinforcement learning algorithm to systematically build optimized quantum circuits for digital quantum simulation upon imposing a strong constraint on the number of allowed quantum gates. With this we consistently obtain quantum circuits that reproduce physical observables with as little as three entangling gates for long times and large system sizes. As concrete examples we apply our formalism to a long range Ising chain and the lattice Schwinger model. Our method makes larger scale digital quantum simulation possible within the scope of current experimental technology.