Online Seminar: Dominik Hangleiter, Freie Universität Berlin
Quantum versus classical learnability of discrete distributions.
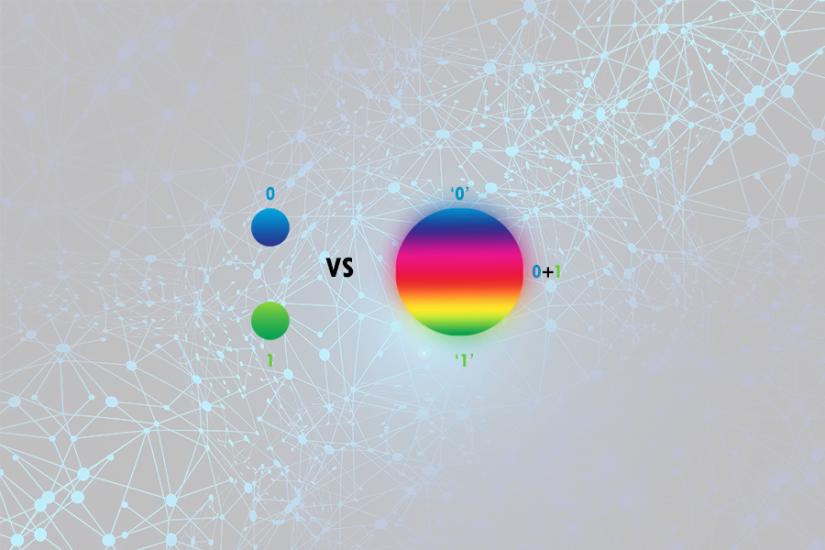
Quantum vs Classical Learnability of Discrete Distributions
SPEAKER: Dominik Hangleiter
AFFILIATION: Institute of Theoretical Physics, Freie Universität Berlin (Free University of Berlin), Germany
HOSTED BY: Dr Márika Kieferová, UTS Centre for Quantum Software and Information
ABSTRACT:
Quantum machine learning has been hailed as one of the promising near-term applications of small quantum computers and much research is focused on devising quantum heuristics that might yield an advantage over classical learning algorithms.
In this talk, we will take a step back and ask: Can we hope for a provable quantum advantage in machine learning? To this end we focus on the following learning task: Given samples from some unknown discrete probability distribution, output an efficient algorithm for generating new samples from that distribution.
Indeed, many machine learning tasks can be reduced to such generative learning of discrete distributions. But it is not at all clear whether or not discrete distributions admit a structure that can be exploited by quantum computers. Our main result is a positive answer to the above question: We explicitly construct a class of discrete distributions which, under the decisional Diffie-Hellman assumption, is provably not efficiently learnable by a classical generative modelling algorithm, but for which we construct an efficient quantum learner.
From a bird's eye perspective, our proof leverages the power of quantum computers to solve the hidden subgroup problem to a distribution learning setting. But we will also take on the mole's perspective and work through an intricate cryptographic argument that proves the (conditional) learning separation.