Our research centres
UTS has research centres that work across disciplines such as health, biomedical, artificial intelligence, data science, robotics, sustainability and water.
Explore
Health and science
Our health and science research advances knowledge and improves outcomes in the medical and scientific fields.
Australian Institute for Microbiology and Infection
Discovery and applied research to build an understanding of how microbes grow, live, adapt and survive, with impact in the areas of antibiotic resistance, food safety and infectious disease.
Centre for Forensic Science
Gathering academics and industry partners who share the vision that crime reduction, crime solving and national security are important for society.
Human Performance Research Centre
World-leading researchers in the areas of sport, exercise science and human performance.
Australian Stuttering Research Centre
Improving the public health problem of stuttering globally.
Human Performance Research Centre
World-leading researchers in the areas of sport, exercise science and human performance.
Australian Institute for Microbiology and Infection
Discovery and applied research to build an understanding of how microbes grow, live, adapt and survive, with impact in the areas of antibiotic resistance, food safety and infectious disease.
Australian Stuttering Research Centre
Improving the public health problem of stuttering globally.
Centre for Forensic Science
Gathering academics and industry partners who share the vision that crime reduction, crime solving and national security are important for society.
Sustainability
Our research creates lasting impact by addressing the complex challenges presented by sustainability.
Climate Change Cluster (C3)
Delivering impactful research to inform solutions to mitigate climate change impacts on our plant-microbe ecosystems, which are critical for the well-being of the animals, plants and our communities.
Jumbunna Research
Producing high quality research on Indigenous legal and policy issues of importance to Indigenous people, their families and communities, and developing highly-skilled Indigenous researchers.
Centre for Clean Energy Technology
Developing efficient devices for energy harvesting, storage, and conversion.
Institute for Sustainable Futures
Creating change towards sustainable futures by conducting independent project-based research for Australian and international clients.
Centre for Clean Energy Technology
Developing efficient devices for energy harvesting, storage, and conversion.
Climate Change Cluster (C3)
Delivering impactful research to inform solutions to mitigate climate change impacts on our plant-microbe ecosystems, which are critical for the well-being of the animals, plants and our communities.
Institute for Sustainable Futures
Creating change towards sustainable futures by conducting independent project-based research for Australian and international clients.
Jumbunna Research
Producing high quality research on Indigenous legal and policy issues of importance to Indigenous people, their families and communities, and developing highly-skilled Indigenous researchers.
Technology and design
Our technology and design research centres develop innovative solutions to the challenges of the modern world.
Robotics Institute
Internationally-acclaimed robotics research group creating positive change for government, industry and the wider community.
Global Big Data Technologies Centre
International centre of excellence for the development of enabling technologies for big data science and analytics.
Transport Research Centre
Global leaders in smart transportation systems and critical transportation infrastructure in modern complex environments.
Global Big Data Technologies Centre
International centre of excellence for the development of enabling technologies for big data science and analytics.
Robotics Institute
Internationally-acclaimed robotics research group creating positive change for government, industry and the wider community.
Transport Research Centre
Global leaders in smart transportation systems and critical transportation infrastructure in modern complex environments.

Business and law
Our Business and law research centres examine how these disciplines shape society through influencing decision-making in the global economy.
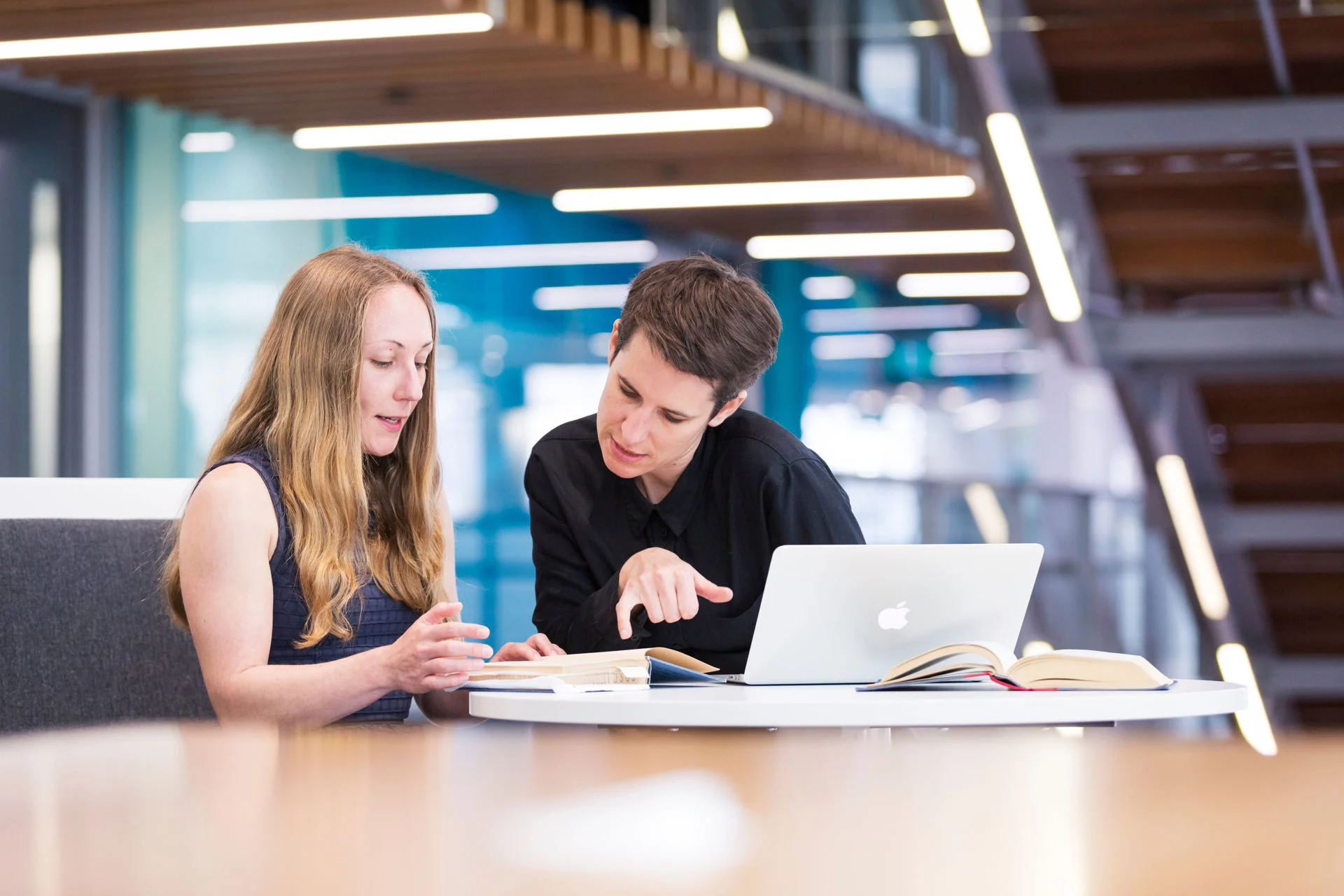
Politics and society
Our politics and society research centres examine the issues affecting governance, public policy, and social change.