Predicting and detecting hate speech online
A new multi-task learning (MTL) model can accurately and consistently detect hate speech on social media platforms.
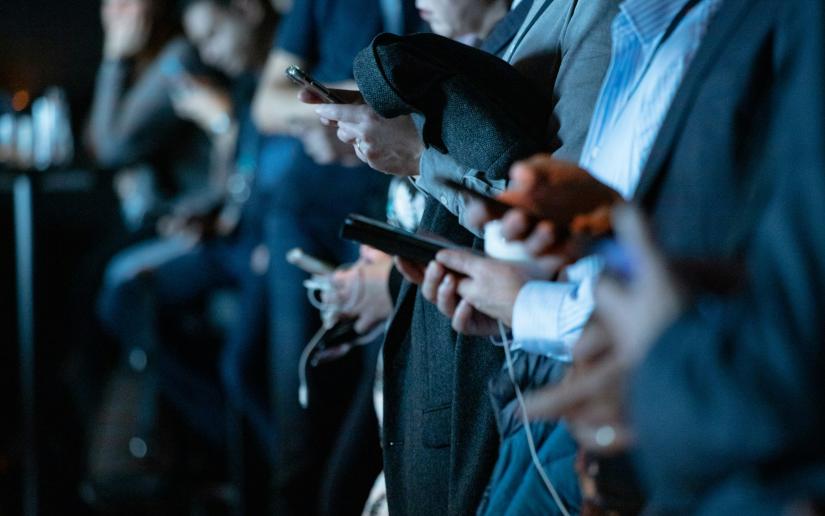
Picture: Camilo Jimenez, Unsplash
Associate Professor Marian-Andrei Rizoiu is leading the way in combatting online misinformation and hate speech. As Head of the Behavioural Data Science Lab at the University of Technology Sydney (UTS), Associate Professor Rizoiu is combining both computer and social science to better predict hateful speech.
Co-authored with UTS PhD candidate Lanqin Yuan, Associate Professor Rizoiu’s research outlines a new automatic detection model in the paper, Generalising Hate Speech Detection Using Multi-Task Learning: A Case Study of Political Public Figures.
A multi-task learning model can perform multiple tasks simultaneously and share information across datasets. In this research, the model was trained on eight hate speech datasets from various platforms including X (formerly Twitter), Reddit, Gab and the neo-Nazi platform Stormfront.
“Designing effective automatic detection of hate speech is a significant challenge. Current models are not effective in identifying all the different types of hate speech, including racism, sexism, harassment, incitement to violence and extremism.”
Associate Professor Rizoiu’s model, however, was able separate abusive language from hate, identifying topics including Islam, women, ethnicity and immigrants. When tested on a dataset of tweets from 15 American public figures, the multi-task learning model was able to identify that out of 5299 abusive posts, 5093 were created by right-leaning figures.
As social media becomes a significant part of our daily lives, automatic identification of hateful and abusive content is vital in combating the spread of harmful content.
Associate Professor Marian-Andrei Rizoiu.