Right-leaning political figures fuel online hate
Researchers have developed a new way to automatically detect hate speech on social media platforms more accurately and consistently using a new Multi-task Learning (MTL) model; a type of machine learning model that works across multiple datasets.
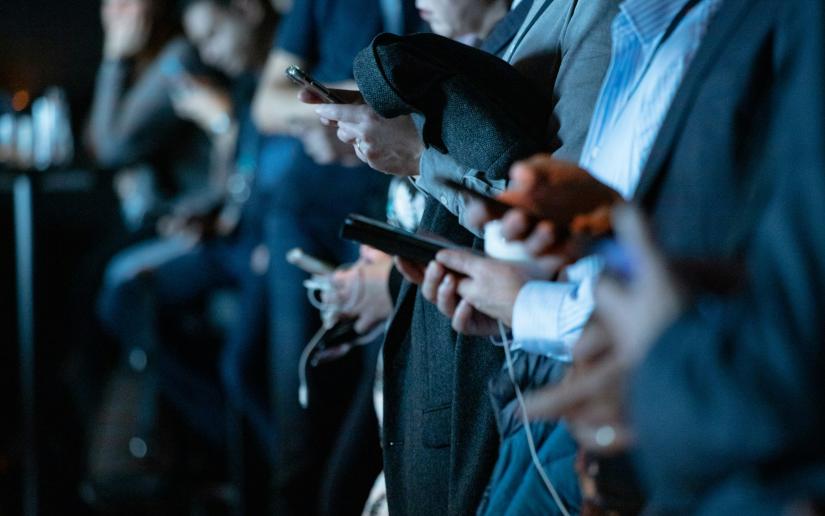
Picture: Camilo Jimenez, Unsplash
The spread of abusive hate speech online can deepen political divisions, marginalise vulnerable groups, weaken democracy and trigger real-world harms, including an increased risk of domestic terrorism.
Associate Professor Marian-Andrei Rizoiu, Head of the Behavioural Data Science Lab at the University of Technology Sydney (UTS) is working on the frontline in the fight against online misinformation and hate speech.
His interdisciplinary research combines computer and social sciences, to better understand and predict human attention in the online environment, including the types of speech that influence and polarise opinion on digital channels.
“As social media becomes a significant part of our daily lives, automatic identification of hateful and abusive content is vital in combating the spread of harmful content and preventing its damaging effects,” said Associate Professor Rizoiu.
“Designing effective automatic detection of hate speech is a significant challenge. Current models are not very effective in identifying all the different types of hate speech, including racism, sexism, harassment, incitement to violence and extremism.
“This is because current models are trained on only one part of a dataset and tested on the same dataset. This means that when they are faced with new or different data, they can struggle and don’t perform consistently.”
Associate Professor Rizoiu outlines the new model in the paper, Generalizing Hate Speech Detection Using Multi-Task Learning: A Case Study of Political Public Figures, published in Computer Speech & Language, with co-author and UTS PhD candidate Lanqin Yuan.
As social media becomes a significant part of our daily lives, automatic identification of hateful and abusive content is vital in combating the spread of harmful content and preventing its damaging effects
Associate Professor Marian-Andrei Rizoiu
A multi-task Learning model is able to perform multiple tasks at the same time and share information across datasets. In this case it was trained on eight hate speech datasets from platforms like Twitter (now X), Reddit, Gab, and the neo-Nazi forum Stormfront.
The MTL model was then tested on a unique dataset of 300,000 tweets from 15 American public figures – such as former presidents, conservative politicians, far-right conspiracy theorists, media pundits, and left-leaning representatives perceived as very progressive.
The analysis revealed that abusive and hate-filled tweets, often featuring misogyny and Islamophobia, primarily originate from right-leaning individuals. Specifically, out of 5299 abusive posts, 5093 were generated by right-leaning figures.
“Hate speech is not easily quantifiable as a concept. It lies on a continuum with offensive speech and other abusive content such as bullying and harassment,” said Rizoiu.
The United Nations defines hate speech as “any kind of communication in speech, writing or behaviour, that attacks or uses pejorative or discriminatory language concerning a person or a group based on who they are,” including their religion, race, gender or other identity factor.
The MTL model was able to separate abusive from hate speech, and identify particular topics, including Islam, women, ethnicity and immigrants.