UTS researchers develop AI system for monitoring hen health
UTS researchers have developed a world-leading artificial intelligence based system to monitor the movement and behaviour of cage-free hens to improve their health and welfare.
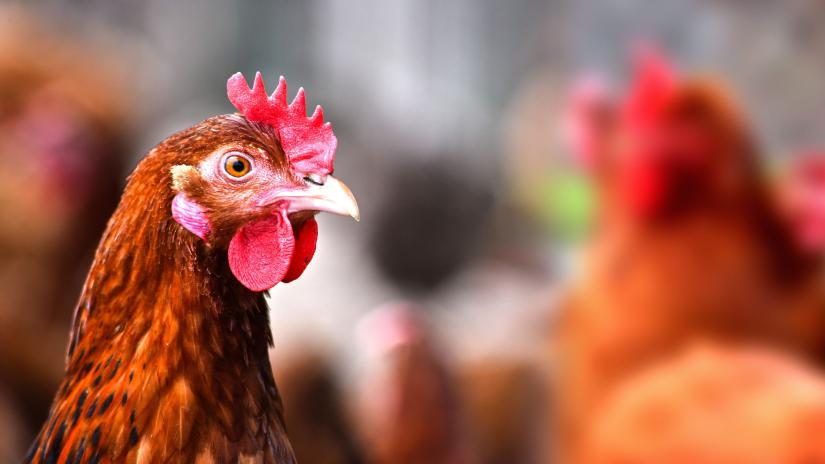
Image: Adobe Stock
Many Australians are big consumers of free-range eggs. They like their hens to roam free on green pastures, or at least large barns, rather than being kept in cages. However, this freedom can also result in increased dangers to hen health.
Researchers from the University of Technology Sydney (UTS) have developed a world-leading artificial intelligence based system to monitor the movement and behaviour of cage-free hens to improve their health and welfare, and minimise the dangers.
Professor Jian Zhang and his team from the Multimedia Data Analytics Lab in the Global Big Data Technologies Centre, based at UTS Tech Lab, are working to develop a real-time video monitoring system, which was recently trialled on an egg farm in Windsor, NSW.
The project is funded by Australian Eggs, a member owned not for profit organisation that invests farmer levies and public funds in research and development on behalf of the egg industry.
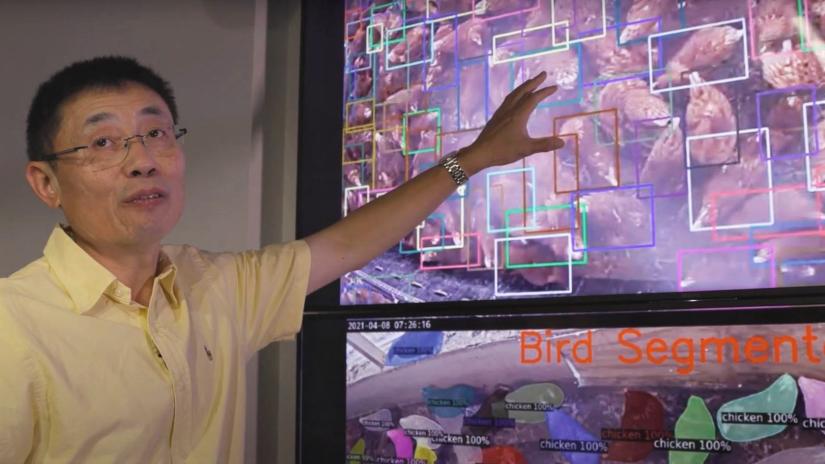
Professor Jian Zhang with the AI hen monitoring system.
“The farm in this project that is trialling the technology has an average of 8,000 hens spread across multiple sheds, and it’s difficult for farm staff to continuously monitor hen behaviour,” Dr Zhang said.
“Cage-free hens have greater freedom to roam and forage, however there is a risk that they can flock together and ‘pile-up’, which can result in hens being smothered. While it is not common, if a pile-up does occur, currently there are no warning system to alert farmers that this might be occurring,” he said.
Effective controls and predictors of risk are not known, so the egg industry is looking to develop effective reduction strategies. Additional to the development of the real-time monitoring system, Australian Eggs has funded a research project that is currently underway to understand what these risk factors are.

We're at an early stage in the journey, we're trying to identify the factors that are happening before pile-ups and smothering, but once we identify those risk factors, we can then program it into the software so that the farmer gets an alert and knows what to do.
Or even better, the program itself can actually start the initiation to prevent them. For example, start the feeders or turn the misters on.
The aim of the project was specifically to look at what are the factors that contribute to bird pile-ups and smothering, and that's why we use this shed who had a couple of experiences in the past.
So we can do that. Better detection, better tracking in the shed and also the bird segmentation image base. So the aim is to do lots of bird behavior analysis.
The ability of the vision system to identify an individual bird and then to track its direction and rate of movement and then leading into density estimates, and finally into an algorithm that can say I think this bird is eating, is drinking, this bird is nesting, etc. We now have all the tools to look for what are the factors that are contributing to pile-ups and smothering.
The benefit of these technologies will help the farm to quickly identify where the pile-up, where the high density, will create a negative impact to bird production.
It's important because what we're looking for are the factors that are leading up to these events, because if we have the factors that are leading up to the events, we can take actions to stop them from happening. And to do that requires 24/7 monitoring and identifying the risk factors and therefore a video based system that is automated with an SMS based system to the farmer is the ideal way to do that.
Okay, from a technology point of view, we first needed bird detection in the image of the video. So this boundry box reflects each bird that has been detected. When we do this detection. Then we move to the next panel. That is you can see is all the birds that will be tracked. Each individual bird will be tracked one by one and you can see the number that is marked for each bird. We roughly understand how many birds and whether they are moving in this scene. So the segmentation means we can do the each individual analysis about the birds behavior, for example, if they are feeding or drinking or even other things. This is very important for the welfare analysis or the production. So this panel actually is more used as an AI model to the density counting. We want to understand in this very small area, whether the birds pile-up. If they pile-up then this is the biggest signal whether they will eventually become smothered.
So what we are trying to do is give farmers a management tool that they can monitor their flock. You saw back at the laboratory that the technology is developed to the point where it can recognize individual birds. You can also track the direction of movement and the velocity. Combined, we can look at the density of those birds. And finally on that last screen, we saw that it was able to recognize individual bird behaviors. With a camera based system you can monitor all birds in this flock that are in the frame of vision for all those aspects. And they will relate to the welfare status of the bird. And over time we'll start to learn the patterns of factors - management environment, etc. that contribute to the birds welfare state and their production.
What we want to know now the next stage is what are the patterns of these coordinated behaviors in the flock? What percentage of the birds are nesting? What percentage of the birds are grooming? What percentage of the birds are hanging around the watering points? And therefore, those patterns may tell us something that we can use to help management - optimal times for feeding for example, what are the factors that are likely to positively influence welfare? And even what are the best ways to manage the internal environment there may be energy savings etc To do that, we don't know these flock coordinator behaviors yet and that's the next stage but using the base technology from this study, to go to that level, which is to say what is the flock doing in the shed that's the next point in the journey.
So what we're looking for, for the farmers, is not for them to have to sit and watch the video 24/7. We are envisaging a system whereby the computer will work out what are the risk factors say for density levels getting to the point that we may have a pile-up, and that will then send an SMS warning to the farmer who's doing other things to say there could be something happening in shed two, come and have a look down the back. But the objective of all of this work is to solve a real problem of farmers take the technology and apply it to solve the problem. And that's what we hope to be doing here.
The new system alerts farm staff to any crowding behaviour, as well as monitoring the hens’ access to food and water to observe changes in behaviours, and any lack of movement in individual hens, which could indicate an injury or illness.
Rowan McMonnies, Managing Director of Australian Eggs, said it was exciting to see a solution that could reduce labour requirements, minimise human intervention in sheds and improve animal welfare.
“This important investment in AI-technology can not only lead to happier, healthier and more productive hens, but also improve farm management and reduce costs,” Mr McMonnies said.
The system currently being trialled uses four cameras: two inside and two outside the sheds. The system has been designed to be scalable and can be increased depending on the area. The technology incorporates pattern recognition algorithms to count and monitor flock density and behaviour.
The UTS team includes poultry and animal behaviour specialists to help analyse and interpret hen behaviour. The next phase of the project will include a deeper analysis of behaviours such as drinking, feeding, foraging and standing.
“Poultry are very sociable and gregarious animals, so we need to build an ethogram, or catalogue of behaviours, which includes both individual and interactive behaviours, to better monitor growth and welfare,” Dr Zhang said.
There are also plans to build a mobile phone app that can alert supervisors to potential trouble. The app will include machine learning technology to improve the system with feedback from users.
The UTS team will run a larger trial across multiple farms in the coming months. Once the trials are complete they hope to commercialise the technology so that it can be implemented on a wider scale. There is also potential to expand the technology to other areas of farming.