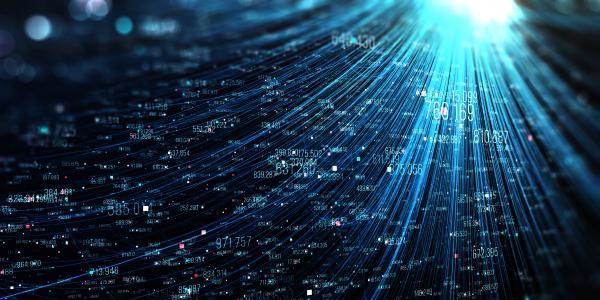
Deep Learning explained
Express your interest
Deep learning has significantly improved classification accuracy for supervised image recognition tasks. However, these tremendous performances are reported on test sets which are drawn from distribution identical to their training datasets.
Evaluating the performance of classifiers with error rate is necessary but not sufficient, since it is agnostic to real-world complexities like open-set and dataset bias. Recently several solutions have been proposed which proposes corrections while training models to reduce such disparate performances.
Some of the goals associated with the project include:
- Identify the sources of bias in face-based gender recognition.
- Empirically estimate the effects of sample selection bias on deep learning models.
Learning opportunities
- Knowledge of Deep Learning – Tensorflow (not Keras)
- Knowledge of Machine Learning
Applicable knowledge
This project is suitable for 4th year students.
How many places are available?
There are two (2) student positions available.
Why is this important?
This project is in line with multiple industry-led initiatives around the ethical use of AI in society, it will also contribute towards UTS’s core goal of the research of international significance.
Project leaders
-
Postdoctoral Research Fellow