Tracking infectious diseases in real time
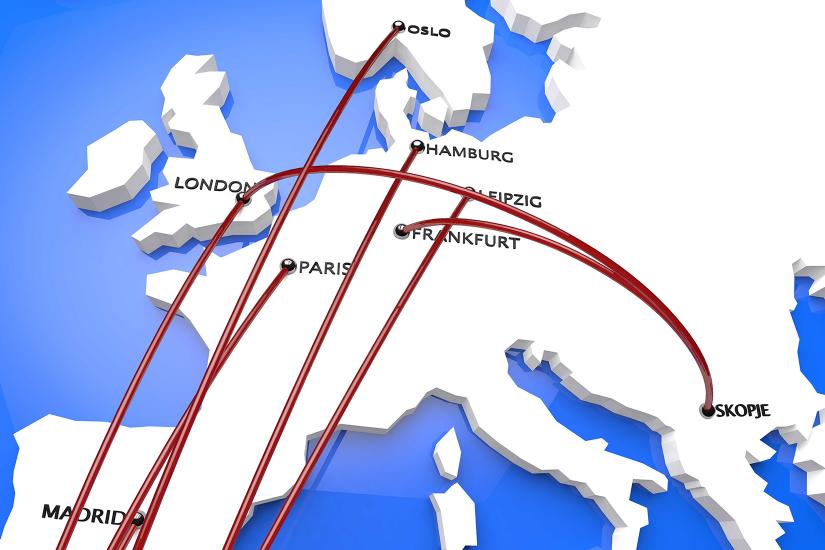
New UTS research has potential to help authorities make more informed decisions about restricting trade and closing borders during infectious disease outbreaks.
Using molecular approaches such as genome sequencing to reveal the complete DNA make-up of an organism has revolutionised the way infectious disease outbreaks are monitored and managed globally. In principle, this genomic information gives authorities unprecedented information about the origin and future trajectory of deadly bugs. However, the robust statistical methods needed to analyse this data haven’t kept pace with advances in genome sequencing.
This may be about to change. Scientists from the University of Technology Sydney (UTS) ithree institute and the US based Fred Hutchinson Cancer Research Centre say they have developed a new algorithm that proves it is possible to complement this molecular revolution with the near real time evolutionary analysis of genomic sequence data collected from infected patients, plants, foodstuffs and waterways.
The new algorithm is able to update an existing analysis rather than starting from scratch.
The research findings, published in two papers in the journal Systematic Biology, open up the possibility that authorities could make much faster and more informed decisions about restricting trade, closing borders, implementing food recalls and allocating resources.
The bioinformaticians devised a new method that can analyse infectious disease data in real time. They showed that not only does the method give “the right answer” via mathematical proof but that the method can also be successfully implemented in practice.
After five years of wrestling with the problem the research outputs are timely. An increasingly interconnected world and the spectre of antimicrobial resistance is challenging disease control centres to stay one step ahead of pathogens that don’t respect borders, the researchers say.
“The problem isn’t the lack or type of data. Public Health laboratories can now sequence pathogens such as Ebola or Salmonella in a matter of days and this vital information is added to already very large databases. The problem is in the way the software analyses the data, the algorithms used” says Associate Professor Darling from the UTS ithree institute.
“Current algorithms can take weeks to run on realistic datasets whereas the window of opportunity to manage an outbreak is often just days,” Associate Professor Darling says.
Current algorithms can take weeks to run on realistic datasets whereas the window of opportunity to manage an outbreak is often just days
In the US the FDA GenomeTrakr initiative for foodborne pathogens alone regularly adds 5000 new genome sequences every month, and now has more than 150,000 sequences in its publicly available database.
“When new genomic data is added [to the database] the previously seen data points needed to be re-analysed. As you can imagine this slows things down considerably and this is costly in both human and economic terms,” Darling says.
Co-author Dr Frederick Matsen says the team’s approach “infers the evolution of pathogens by simulating an evolutionary process on the solutions themselves”.
“The solutions compete with each other to reproduce, with their reproductive advantage being proportional to how well they explain the data. So we are using evolution on the computer to learn about evolution in nature,” Dr Matsen says.
Lead author and UTS ithree institute senior research fellow Dr Mathieu Fourment says that the recent Ebola and Zika outbreaks demonstrate that “the ability to rapidly and accurately identify the source of an outbreak is critical for containing the spread of the disease”.
“Although Australia has been free of Ebola and Zika, threats such as equine influenza and Dengue occur periodically and climate change may increase the risk of an outbreak. It is crucial that authorities are equipped with a strong surveillance and management framework to protect the public from these threats,” Dr Fourment says.
Publication details:
- Effective online Bayesian phylogenetics via sequential Monte Carlo with guided proposals Mathieu Fourment, Brian Claywell, Vu Dinh, Connor McCoy, Frederick A Matsen IV and Aaron Darling. Systematic Biology (2018) 10.1093/sysbio/syx090
- Online Bayesian phylogenetic inference: theoretical foundations via Sequential Monte Carlo Vu Dinh, Aaron Darling and Frederick a Matsen IV. Systematic Biology (2018) 10.1093/sysbio/syx087