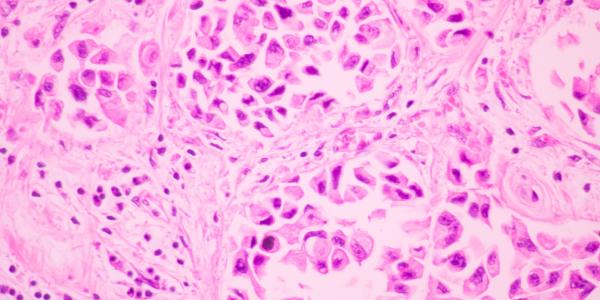
Benchmarking methods explained
express your interest
In the last few years, single-cell transcriptomics (scRNAseq) has become the preferred method to understand the cell composition and function of healthy tissues1 and tumours2, 3 through unsupervised sampling and modelling of transcriptional states in single cells4.
The recent development of highly parallel single-cell RNAseq methods has resulted in a similar advance of analytical methods to explore and visualise high-dimensional data. There are currently available several computational frameworks designed explicitly for scRNAseq analysis5-8 (R environment).
Using several single-cell RNAseq datasets of breast cancer generated in the lab; this project aims to compare different computational approaches to extract biologically meaningful data and visualise various features to annotate and functionally characterise tumour cell diversity. These include quality control and normalisation methods, data integration, dimensional reduction, pseudotime alignment and cell-to-cell communication inference.
Learning opportunities
- Development/implementation of a streamlined computational pipeline for demultiplexing and alignment of single-cell RNAseq analysis.
- Benchmark of quality control and normalisation methods.
- Benchmark of single-cell RNAseq analytical and visualisation tools.
Applicable knowledge
This project is suitable for 3rd or 4th year students with computational skills in Bash/UNIX, R, python and knowledge of statistics.
How many places are available?
There are two (2) student positions available.
Why is this important?
The efficient implementation of flexible and rapid computational methods for single-cell RNAseq analysis is crucial for the development of new approaches for the integration of single-cell multi-omics analysis. Novel methods enable in silico testing of hypotheses and assist to solve technical variation on single-cell genomics data.
Project leaders
-
Associate Prof & Director, Single Cell Genomics Facility